The Ultimate Guide to Labeling Tool Machine Learning
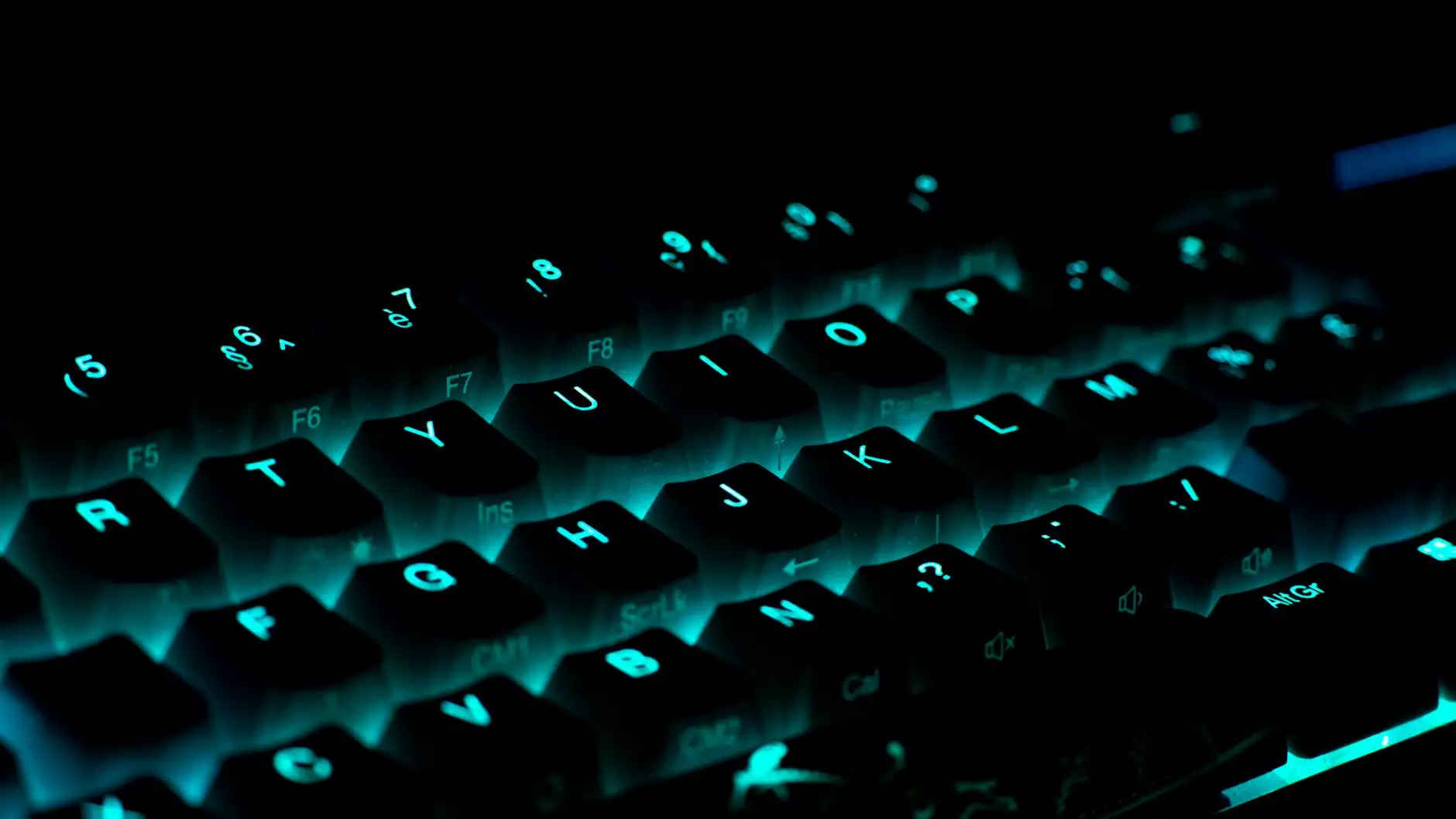
Introduction to Data Annotation
In the realm of machine learning, effective data annotation is paramount. It involves the process of marking up or labeling data to train algorithms to understand and learn from it. Whether you are dealing with images, text, or audio, a robust data annotation strategy is essential. In this article, we will delve into the significance of labeling tool machine learning and how it can transform the way businesses approach data management and model training.
What is a Labeling Tool in Machine Learning?
A labeling tool is an application or software designed to facilitate the annotation of training data for machine learning models. This process is crucial as it directly influences the model's performance. The tool enables users to attach labels to various data types, providing the necessary context for the algorithms to learn. Here are some key attributes of effective labeling tools:
- User-Friendly Interface: An intuitive interface allows users of all skill levels to contribute to the annotation process effectively.
- Customizability: The option to create custom annotation tasks tailored to specific projects enhances the versatility of the tool.
- Collaboration Features: Support for team collaboration ensures that multiple users can contribute to data labeling simultaneously.
- Integration: Compatibility with existing machine learning frameworks and tools is vital for a seamless workflow.
Importance of Data Annotation in Machine Learning
The backbone of any successful machine learning project is its training data. Properly annotated data allows models to learn patterns and make predictions with higher accuracy. Here’s why data annotation matters:
- Enhanced Model Accuracy: Well-labeled data leads to improved predictive accuracy as the model learns to identify correct patterns.
- Reduction of Bias: A diverse and well-annotated dataset helps to minimize biases in machine learning models, promoting fairness.
- Faster Development Cycles: Effective annotation tools streamline the labeling process, reducing the time needed to train models.
How Labeling Tool Machine Learning Improves Efficiency
The fusion of labeling tools and machine learning offers transformative efficiency improvements. Here are some ways that adopting a labeling tool enhances data handling:
1. Automation of Repetitive Tasks
By leveraging machine learning algorithms, labeling tools can automate repetitive tasks in the annotation process. For instance, when labeling images, the software can quickly identify patterns and suggest labels, significantly speeding up the process.
2. Improved Quality Control
With built-in quality assurance features, modern labeling tools can flag inconsistencies in data labeling. This improves the overall quality of the training dataset, ensuring that the machine learning model is built on reliable information.
3. Scalability
Machine learning projects often scale rapidly. Using a robust labeling tool allows businesses to increase their data processing capabilities without compromising quality. This scalability is essential for organizations that want to keep up with evolving technology and customer needs.
Choosing the Right Labeling Tool
When selecting a labeling tool for machine learning projects, businesses should consider several factors:
- Type of Data: Understand the type of data being annotated - whether it’s text, images, or audio - and find a tool that specializes in that area.
- Budget: Evaluate the cost versus the features offered. Some tools may provide extensive features at a premium price.
- User Training: Consider whether the tool provides adequate training resources for users to get started quickly.
- Customer Support: Access to responsive customer support can facilitate problem resolution and enhance user experience.
The Integration of Machine Learning and Labeling Tools
The synergetic relationship between labeling tool machine learning and businesses offers numerous advantages. By integrating machine learning into labeling processes, companies can reap benefits such as:
1. Predictive Analytics
Some labeling tools now incorporate predictive analytics to suggest labels based on previous annotations. This feature not only saves time but also ensures consistency across the dataset.
2. Real-time Collaboration
Modern machine learning platforms often support real-time collaboration among teams. This capability allows for instant feedback and adjustments during the annotation process.
3. Insights through Data Analysis
By analyzing annotated data, organizations can extract valuable insights that can inform business decisions. These insights often lead to innovations and improvements in products or services.
Challenges in Data Annotation
While labeling tools have greatly improved data annotation processes, certain challenges remain:
- Quality Assurance: Ensuring the accuracy of labels remains a challenge, especially with large datasets.
- Labeling Bias: There is a risk that annotators may impose their biases on the data, affecting model outcomes.
- Resource Intensity: Data annotation can still be a resource-intensive process, requiring significant human involvement.
Future Trends in Labeling Tools for Machine Learning
The future of labeling tools in machine learning is paved with exciting advancements that promise to enhance efficiency, accuracy, and user experience:
1. Enhanced Automation through AI
As artificial intelligence continues to evolve, future labeling tools will likely become more autonomous. AI will be able to take on more complex labeling tasks, reducing the dependency on human annotators.
2. Greater Emphasis on User Experience
As competition grows among labeling tool providers, the focus on creating user-friendly interfaces and experiences will expand, making tools accessible to a broader audience.
3. Increased Customization Options
Future tools will also provide even more customization options to cater to specific business needs, allowing organizations to tailor their annotation processes deeply.
Conclusion
In the evolving landscape of machine learning, the role of labeling tool machine learning cannot be understated. As businesses continue to leverage data to drive insights and innovation, the need for effective data annotation solutions will only grow. By selecting the right tools and embracing modern techniques, organizations can not only improve their efficiency but also achieve superior results in their machine learning endeavors.
This guide has explored the pivotal role that data annotation plays in machine learning, highlighted the importance of selecting quality labeling tools, and provided insights into the future of data annotation. For businesses looking to stay ahead of the curve, investing in the right data annotation platform is crucial. Visit keylabs.ai to discover how our innovative solutions can enhance your data annotation processes.